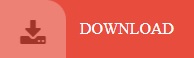
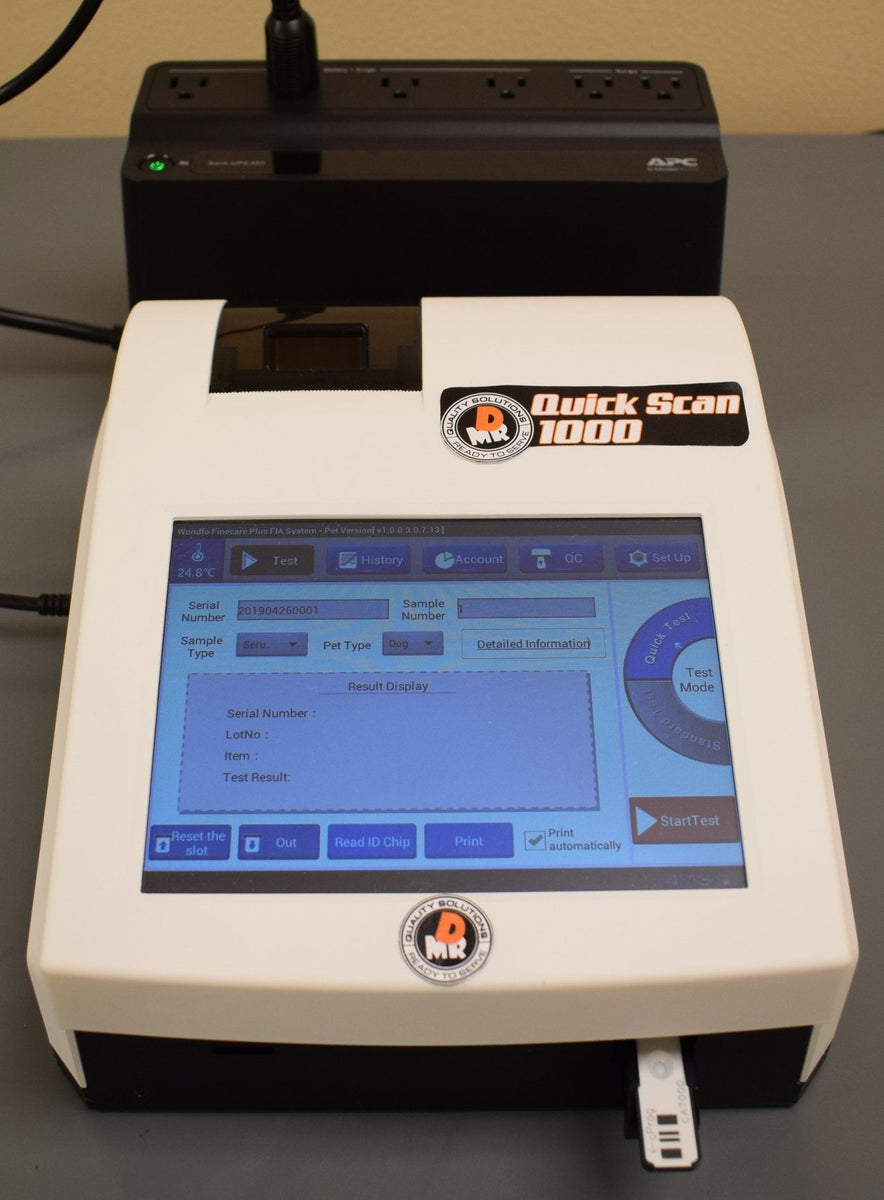
Low voltage up to 10 kA 1-phase, 5 kA 3-phase, and 10 kA DCĪn outdoor live 25 kV distribution system that includes a full-height pole yard, 2 MW / 600 MVA loadbank, reclosers, FCIs, switchgear and full SCADA.
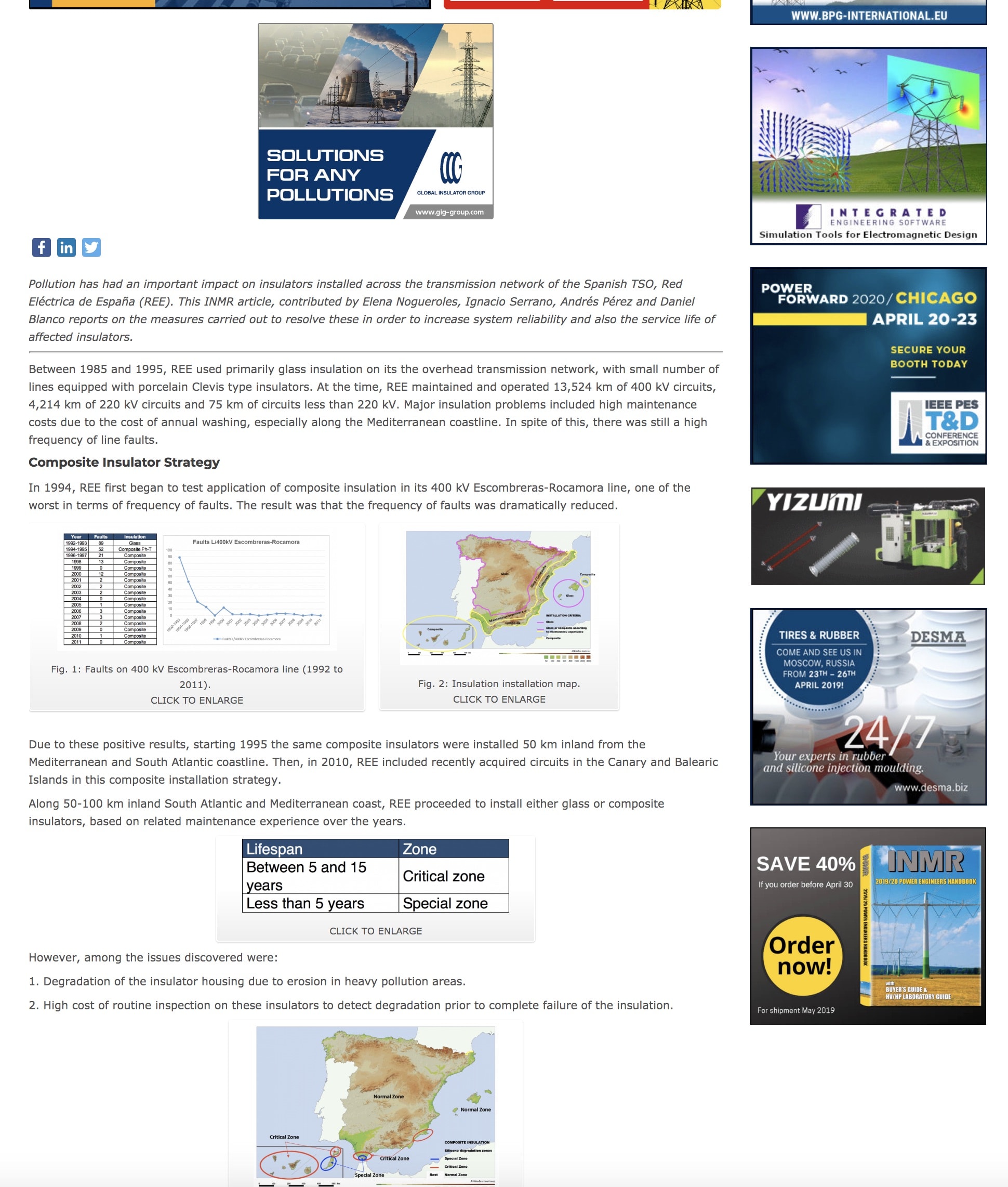
High current tests specified to IEEE C37.60, C37.41, C37.30, C37.74, C37.20, 386, 837, 495 ASTM F81Īrc resistance tests on switchgear cubicles, explosion resistance tests on distribution transformers, power arc tests on insulators, capacitive current interruption tests (new line), arc flash, power equipment failure simulation. Kind of Power Source for the HP Laboratory:ĭirect 230 kV feed from major substation.ģ.7 kV to 39 kV, 3-phase up to 80 kA 3.7 kV to 45 kV, 1-phase up to 100 kA Total Number of Employees in the HP Laboratory:
INMR FROM SCAN ISO
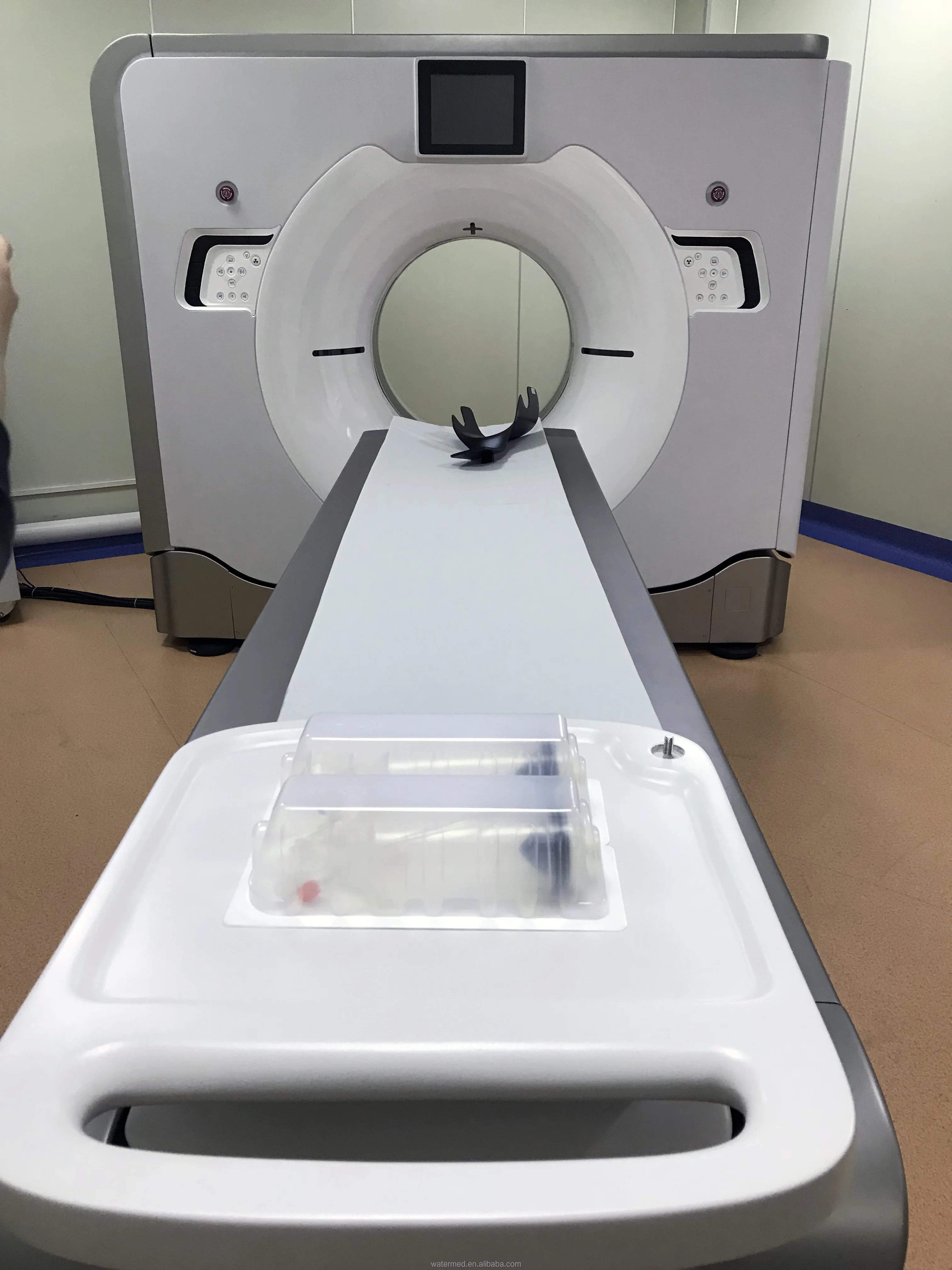
INMR FROM SCAN PORTABLE
High current test thermal test mechanical test mobile testing, radio influence voltage (RIV) condition assessment.ĩ0 kV VFRTS and 800 kV portable 50/60 Hz resonant test set. Other Tests Performed on Insulators, Arresters, Bushings, Cable Terminations: Tracking wheels spray wheel, dip wheel salt fog/ weathering chamber – IEC 61109, IEC TR 62730 and ASTM G53 (40 kV) Testing includes development, qualification, commissioning, and acceptance. High current load cycling is also available up to 3000 A.ġ00 m. Portable variable frequency resonant test set, 90 kV, max. With moredata that can be made available, this may be a reasonable trade‑off where decline in performance may be counter‑acted with more data.Total Number of Employees – the HV Laboratory:ġ5 m length x 6 m width x 2.5 m height Dielectric Testing FacilitiesĦ m length x 6 m width x 7 m height Transformer TestingĬable systems up to 500 kV, including lightning impulse and switching impulse, hipot, and PD measurements. Comparisons with that of using annotated GT tumor data fortraining showed an average of 3.0% degradation (2.92% for 1p/19q codeletion status and 3.23% for IDH genotype).Conclusion: Using tumor ROIs, i.e., ellipse bounding box tumor areas to replace annotated GT tumor areas for train‑ing a deep learning scheme, cause only a modest decline in performance in terms of subtype prediction. Experiments wereconducted on two datasets (US and TCGA) consisting of multi‑modality MRI scans where the US dataset containedpatients with diffuse low‑grade gliomas (dLGG) exclusively.Results: Prediction rates were obtained on 2 test datasets: 69.86% for 1p/19q codeletion status on US dataset and79.50% for IDH mutation/wild‑type on TCGA dataset.
INMR FROM SCAN MANUAL
ellipse shaped boxes) for classification without a significant drop in performance.Method: In patients with diffuse gliomas, training a deep learning classifier for subtype prediction by employ‑ing tumor regions of interest (ROIs) using ellipse bounding box versus manual annotated data. The aim of our study is to see whether it is possible to replace GT tumor areas by tumor bounding boxareas (e.g. Analogous to visual objecttracking and classification, this paper shifts the paradigm by training a classifier using tumor bounding box areas inMR images. However, itdoes not guarantee the quality and could lead to improper or failed segmented boundaries due to differences in MRIacquisition parameters across imaging centers, as segmentation is an ill‑defined problem. As an alternative automatic segmentation is often used. The manual annotation is a time consumingprocess with high demand on medical personnel. However, most of these methods require annotated datawith ground truth (GT) tumor areas manually drawn by medical experts. Recent machine learning and deep learning (DL) approaches may help theclassification/prediction of tumor subtypes through MRIs. Background: For brain tumors, identifying the molecular subtypes from magnetic resonance imaging (MRI) isdesirable, but remains a challenging task.
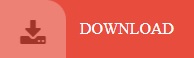